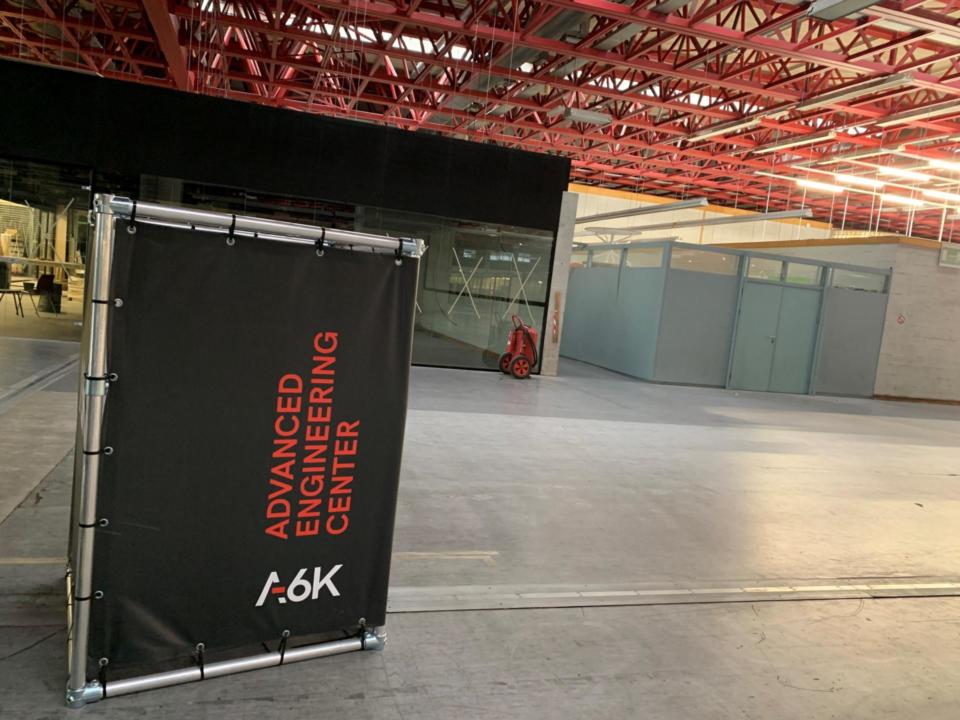
A little over a year ago, the TRAIL initiative was launched to boost AI research and enable it to accelerate this strategic field in the Walloon Region by facilitating the adoption of AI by the socio-economic fabric.
On Friday 6 May, this desire became a reality with the meeting of key industrial players such as AISIN, I-care, Sagacify and other AI user companies, but also AI service providers. This meeting took place in A6K premises. On this occasion, more than 150 people from the academic world, the industrial world and public actors active in this field exchanged on their technological challenges. The aim was to pool knowledge and resources to enable Wallonia to position itself among the recognised AI ecosystems in Europe.
This event was organised under the auspices of the Vice-President of Wallonia and Minister of Digital Affairs, Willy Borsus.