Geraldin Nanfack, a researcher at UNamur, has been working since December 2018 on his thesis as part of the EOS VeriLearn project. His research work aims to ensure that Machine Learning algorithms meet properties or certain constraints. For example, in one of his works [1] published at the ESANN’21 conference, he proposed a method to force decision tree algorithms to make fair decisions with respect to sensitive characteristics such as race or gender. In another work [2] published at UAI’21, he developed a method to force so-called “black-box” and differentiable models such as neural networks to be easily and globally explained by decision rules so that non-expert users of this artificial intelligence can understand how the decisions were made.
His work aims at having a more reliable Artificial Intelligence (AI), which can be endowed with faculties to provide faithful explanations of its behavior. This evolution of AI is valuable for several sensitive sectors such as health or banking that could use an AI predisposed to provide reliable explanations of its decisions while guaranteeing or maximizing fairness in its predictions.
[1] Nanfack, G., Delchevalerie, V., & Frénay, B. (2021). Boundary-Based Fairness Constraints in Decision Trees and Random Forests. In The 29th European Symposium on Artificial Neural Networks, Computational Intelligence and Machine Learning, https://proceedings.mlr.press/v161/nanfack21a.html.
[2] Nanfack, G., Temple, P., & Frénay, B. (2021, December). Global explanations with decision rules: a co-learning approach. In Uncertainty in Artificial Intelligence (pp. 589-599). PMLR, https://pure.unamur.be/ws/portalfiles/portal/61249591/ES2021_69.pdf.
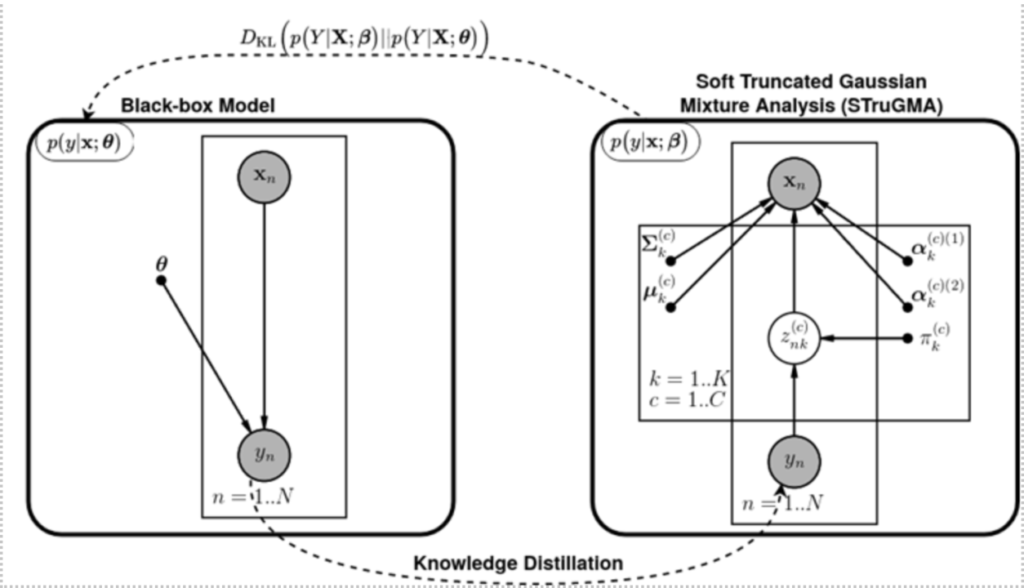