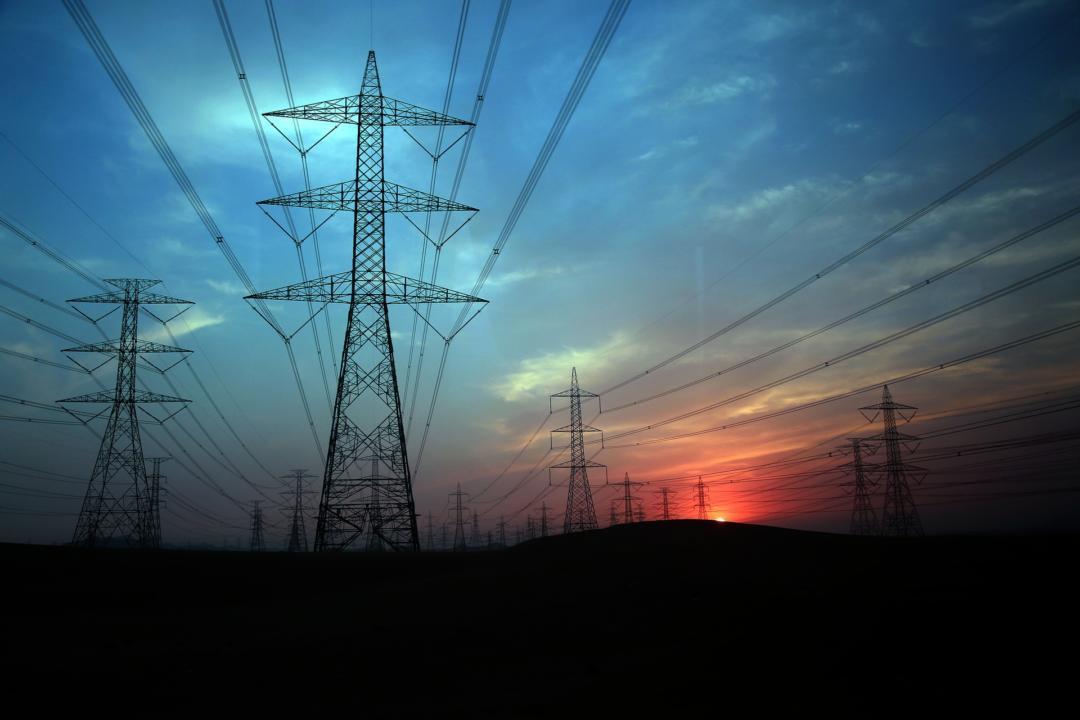
Machine Learning-based Energy Prediction, Management, and Optimisation Towards Better Energy Decision-Making Process
Model-Driven AI
Aim
The aim is to help better understand the energy performance of buildings on a large scale in order to help stakeholders make better decisions when it comes to improving energy efficiency.
What's at stake
The need for a transition towards a low carbon economy to limit the effect of climate change does not only mean increased use of renewable energies, it also implies a more efficient use of the energy that we have avoiding waste. Better managing the energy of our buildings using artificial intelligence goes exactly in this direction. Indeed, buildings are responsible for more than 40% of power consumption and greenhouse gas emissions worldwide. Building energy modelling and forecasting is a key tool to provide quantitative insights for different stakeholders and to inform their decision-making when it comes to energy planning, building design, predictive maintenance, energy standards recommendations, policies definition, and more.
Different sources of “data” could be jointly used for the generation of building energy performance prediction model:
- Data from sensors and smart meters embedded in buildings;
- External and environmental data such as weather measured data and forecasting;
- Simulated data from building energy simulation model.
Challenges
However, the cost of equipping and monitoring buildings with appropriate sensors and meters is not negligible. This is particularly the case in older buildings, which feature the highest energy consumption, but were not designed to incorporate those technologies. Furthermore, historical data are difficult to obtain as this type of monitoring equipment remains relatively recent. Finally, the numerical simulations used to model energy consumption are costly and are not always sufficiently accurate, especially if input data are not available and have to be estimated.
AI possible solutions
Transfer learning is a promising technique to develop accurate and reliable building energy prediction models. It uses the knowledge about energy performance learned from a set of information-rich buildings (e.g., recent constructions) to buildings with limited data (e.g., older housing). Transfer learning strategies could potentially reduce the computational time and cost, while also improving the accuracy of predictions. An exciting challenge for our AI researchers.
Key AI tech topics :
- Transfer learning
- Demand response
- Smart grid modelling