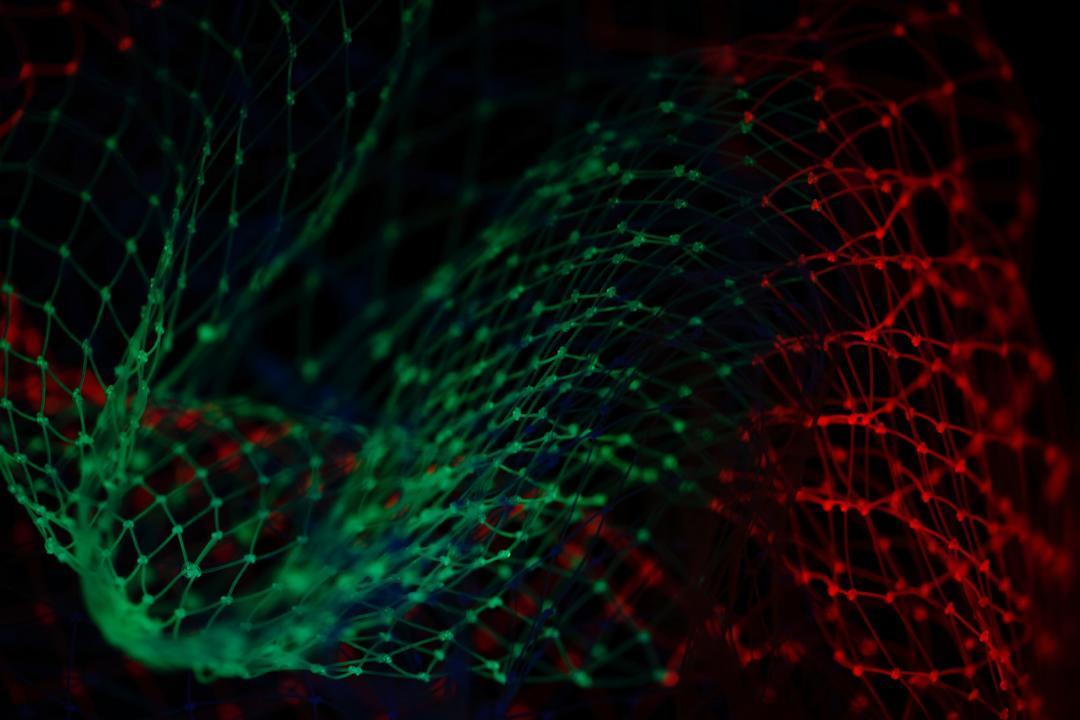
Weakly-Supervised Machine Learning, Towards a More General AI
Embedded AI
Aim
The aim is to create and validate AI solutions that drastically reduce the need for enormous amounts of labelled data and demonstrate strong generalisation capabilities.
What's at stake
In recent years, AI has brought positive contributions in the quality and efficiency of products, sparing of ressources, personalisation of services or improving decision-making. However, there is a limit to what present AI technologies can achieve because they rely on huge amount of data carefully annotated by human experts. Building those datasets is time-consuming and costly which is a brake on the adoption of AI.
Challenges
Labelled datasets exploited by present AI technologies, dominated by supervised learning, require expert human annotation which is an expensive and time-consuming process. It can even prove to be impossible for advanced AI algorithms to generalize to newly discovered contexts since the whole world cannot be annotated. New technologies drastically reducing the need for human-labelled data are mandatory to lower the cost and development time of AI applications. They will foster the adoption of AI and pave the way for more advanced AI technologies.
AI possible solutions
Self-supervised, semi-supervised, few-shot and active learning are promising AI techniques with the potential to make AI solutions perform tasks for which little to no manually-labelled data is available. These methods should reduce the large amount of required annotations and hence minimise human intervention. An exciting challenge for our AI researchers.
Key AI tech topics
- Contrastive and non-contrastive self-supervised learning
- Active learning
- Few sample learning
Get in touch
Anh-Thu PHAN-HO (phanho@multitel.be)